Our central research goal is to understand the evolution and function of the complex molecular networks that underlie life. Our research program thus integrates population genomics and systems biology toward a long-term goal of incorporating molecular mechanisms into evolutionary genetics.
Population genomics
We have unique expertise in numerical diffusion approaches for modeling population genomic data, stemming from Ryan’s development of the software dadi (Diffusion Approximations for Demographic Inference). For example, we developed a diffusion approach for modeling selection on triallelic sites, which we applied to assess the similarity in selection coefficients of different mutations at the same protein site (Ragsdale et al. Genetics 2016). We strive to make our modeling approaches broadly useful to the research community. For example, we showed that the Godambe Information Matrix provides an effective and computationally efficient approach to statistical analysis of population genetics models (Coffman et al. Mol Biol Evol 2016). We also incorporated models of inbreeding into dadi (Blischak et al. Mol Biol Evol 2020).
We often collaborate with others to apply population genomic analyses to interesting data sets. For example, we collaborated extensively with the group of Michael Hammer on the genomics of African Pygmies, infer demographic history and natural selection (Hsieh et al. Genome Res 2016a) and interbreeding with extinct hominids (Hsieh et al. Genome Res 2016b). Other past projects have focused on the human X chromosome (Veeramah et al. Mol Biol Evol 2014), orangutans (Locke et al. Nature 2011 and Ma et al. PLoS ONE 2013), and desert tortoises (Edwards et al. Ecol Evol 2016).
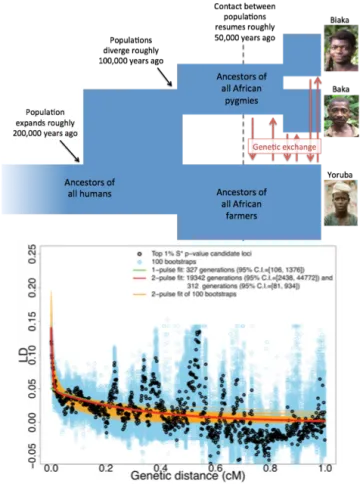
Evolutionary systems biology
Our group also has unique expertise in integrating dynamical systems biology models of molecular networks with genomic data. A particular focus has been predicting rates of protein evolution. Knock-out experiments explain very little of the variation in evolutionary rates. This has led some researchers to doubt whether function plays much of a role in constraining protein evolution. We think knock-out experiments may be misleading, because knock outs are massive perturbations, and many evolutionarily-relevant mutations introduce only small perturbations. We use dynamical systems biology models to assess the effects of differentially small perturbations to molecular functions. Using this approach, we showed that proteins with greater influence on network dynamics evolve more slowly in a large number of networks (Mannakee and Gutenkunst PLoS Genetics 2016) and in yeast pyrimidine biosynthesis (Hermansen et al. BMC Evol Biol 2015).
Our modeling work is complemented by bioinformatic approaches. For example, we recently analyzed all human and yeast proteins in terms of solvent accessibility, structural disorder, expression level, and mutation frequencies to test the strength of selection against spurious phosphorylation by tyrosine kinases (Pandya et al. Mol Biol Evol 2015).
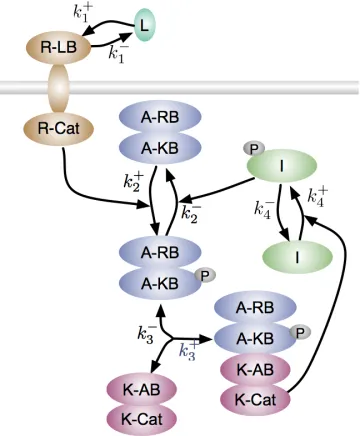
Cancer
Cancer unifies evolutionary and systems biology, because tumors begin when mutations disrupt cellular networks to confer a growth advantage on particular cells. Cancer is thus an increasing topic of research in our group. For example, we recently developed a mathematical model to investigate the role dedifferentiation may play in stem-cell driven cancers.We found that de-differentiation acts as an additional selective advantage for cancer stem cell populations, and that loss of stem-cell homeostasis allows de-differentiated cells to accelerate tumor formation (Jilkine and Gutenkunst PLoS Comput Biol 2014). These findings suggest that preventing de-differentiation may be therapeutically important.
We also apply population genomic insights to the study of cancer. For example, we developed a novel approach for identifying somatic mutations from sequence data that leverages the biological fact that each tumor has a unique mutation profile (Mannakee and Gutenkunst NAR Genomics and Bioinformatics 2020).
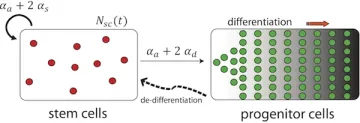